Can machines learn? RGA Senior Data Scientist Dr. Dihui Lai thinks so, and he should know. Dr. Lai has devoted his career to studying the application of artificial intelligence to insurance – particularly to facultative underwriting.
With each facultative application, highly skilled underwriters must painstakingly evaluate complex and sometimes conflicting pieces of information using comprehensive knowledge and nuanced judgement. Such a review cannot be mirrored by a machine. On the other hand, those same facultative underwriters must also perform repetitive, often tedious, tasks. The key question now facing insurers: How can they best leverage new data sources, predictive modeling and machine learning to further automate the underwriting process and increase the efficiency of facultative underwriters?
Lai is working on the answer. Fueled by data and powered by AI, machines such as “deep neural networks” have learned to carry on conversations, drive vehicles, defeat chess grand masters, paint pictures and predict the future. So why not underwriting? Lai recently unveiled an RGA-developed “AI-Augmented” underwriting system at the Society of Actuaries Predictive Analytics Symposium and took a few moments to explain the results.
Artificial intelligence, Deep Learning, and Machine Learning are the buzzwords of the moment. How much of this is hype – and what are the real-world implications for underwriting?
The term artificial intelligence or AI is used – and misused – a lot. It’s definitely a buzzword, and yet many of the people who are talking about artificial intelligence might not fully understand it. Computers are really great at repetition, and AI is about using repetition to recognize patterns and adjust to them – to learn without being directed at every step. Consider Google’s AlphaGo AI, which defeated a world champion player of the Asian game of Go. The system’s machine learning uses big data to teach a computer to recognize patterns, and the computer can then make strategic choices by itself. It sounds impressive, but AI is actually very preliminary – the technology is still at its infant age.
Andrew Ng of Stanford University [Google Brain co-founder and computer scientist] set forth a rule of thumb to describe AI’s current state. He says AI technology today has the capability to solve the same problems a human can solve in three seconds. AI can recognize a voice or a face to unlock the newest iPhone, but Apple’s smart virtual assistant Siri still seems pretty naive.
So, there is no super-intelligent computer that could solve everything. The way I see it, AI today is at a similar stage to where the Internet was in the 70s. I can still remember trying to connect using telephone lines and slow modems. Remember the beeping! Who could have imagined the amazing connection speeds and live streaming we have today? Similarly, right now AI applications are limited. We are just at the beginning.
Bringing the conversation back to insurance, you recently spoke to the Society of Actuaries about the application of AI to facultative underwriting. Can you explain what you were looking to achieve?
First, it’s important to say that underwriting is extraordinarily complex; no AI technologies available today could fully underwrite a facultative application. But what we do have are multiple “AIs” – multiple voice, image, face, character and translation algorithms that we can stack like building blocks to support underwriters.
Our team set out to see if we could build a solution to enhance underwriting efficiency – what we call an “AI Augmented Underwriting System” solution stack. We began by working closely with underwriters to identify their needs. We looked at the “workflow” or process for a typical underwriter from the perspective of a data scientist. What types of data must be reviewed? PDF images? Tables? Text? XML files?
We learned that one of the most common challenges was the time-consuming manual review of PDF images, requiring both visual review and transcribing work. If you think about each task the underwriter is trying to accomplish as different functions of a brain, this makes sense. You use your eyes to read the page – that’s optical character recognition or OCR, a form of artificial intelligence that can find patterns in images. You read and there is natural language processing, which can mine text for sequences of words. We break this down to independent tasks, with each having an AI piece to help or augment what the underwriter is doing. That’s the stack.
Our AI Augmented Underwriting system uses OCR, natural language processing, other machine-learning and even rule-based algorithms to solve a specific problem – how to make the underwriter’s job easier.
Well, if a robot can’t replicate facultative underwriting, then what is the purpose of this AI augmented system?
There are so many things you can do with AI to increase the efficiency of the underwriting process. Our system takes in unstructured data and analyzes questionnaires, names, motor vehicle records and prescription histories; locates signatures; classifies pages and sorts and analyzes attending physician statements. This information is then provided to the underwriter, who reviews this output and makes a decision.
Take our Page Classifier. A facultative case application can reach into the thousands of pages the underwriter must scan. Imagine if AI could do that instead? Our page classifier can, for example, scan a huge case file and note that on page 270, the underwriter will find APS information and on page 280 the underwriter will find medical impairments that might cause concern. The same goes for our system’s Consistency Check. Sometimes an applicant or agent might insert wrong pages, randomly mixing in another applicant’s information. AI could help the underwriter spot these faster. 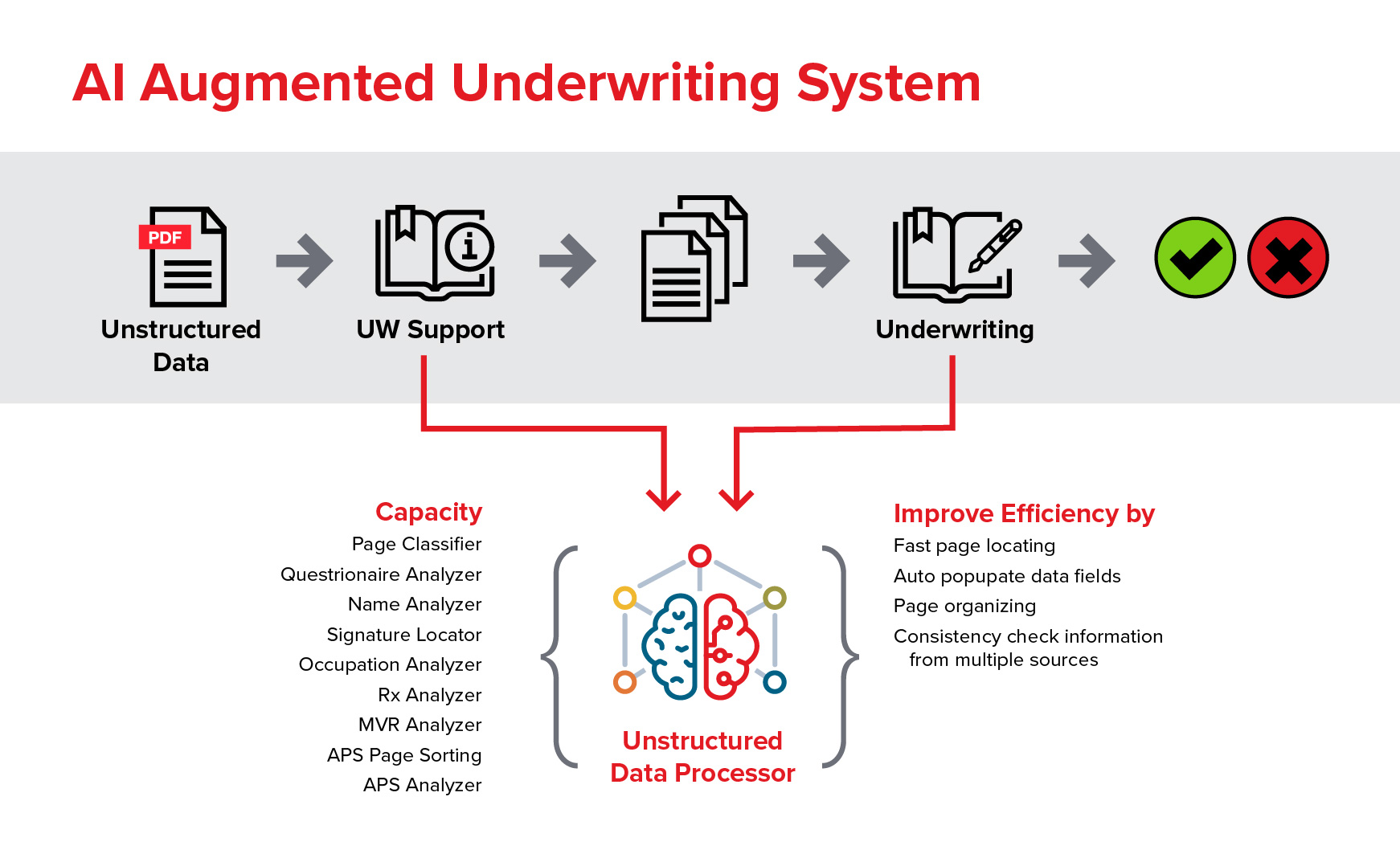
Unpack the efficiency argument a bit. What advantages did you identify?
Faster page locating, auto-population of data fields, an automated search for inconsistent and contradictory information within an application. Such information can include two dates of birth, ICD code extraction to quickly identify severe medical conditions, page organizing – the list goes on.
Our goal is not to replace the underwriter – it is to free the underwriter from repetitive, mundane tasks so that he or she can focus on the decision. And we met that goal. We built the system, and it works. It is a really exciting time.
Exciting but imperfect. In your presentation, you caution that the quality of outcomes relies on a data set that is of consistent format and decent quality. So are you saying garbage in, garbage out?
Yes. That’s why we call this an augmented system. We are augmenting the facultative underwriter’s role, not replacing it. Think about it. Underwriting, especially facultative underwriting, is an extremely complex problem. Facultative cases, almost by definition, have inconsistencies and nuances to process as well as knowledge on complex medical conditions to apply in order to assign a class. No AI technologies today can accomplish this level of cognition right now.
Also the AI can attempt to read a physician’s handwriting in a scanned APS (Attending Physician Statement), but if a human can’t make sense of the scribble and has to guess at the meaning, an AI system is not going to succeed.
Our system merely can more efficiently point out patterns – not assess their implications. Nor can we tackle fundamental AI problems or compete with giants such as Google, IBM or Intel.
What do you mean?
There is no chance we would compete with Google and other companies that specialize in data science. But the issues insurers and underwriters face are unique. We have problems that people at IT companies like Google are not looking to solve – and may not even know exist.
That’s where RGA comes in – we are looking to bridge this gap, to go one layer deeper and look at the technology behind AI and machine learning. The fundamental problem of AI is how to build machines that are curious, that learn from mistakes. For example deep learning, a form of AI that requires minimal guidance, but ‘learns’ as it goes. This relies on algorithms you could adapt for different purposes. We can identify these building blocks, and put them together to solve specific insurance problems. There is no doubt that there is huge market demand. The challenge is finding the right method and the right people who have interdisciplinary knowledge across AI and insurance, particularly life and health cover.
In logistics, we want inventory to be moved and organized. In transportation, we want autonomous vehicles that can navigate. Why can’t we also turn to machines in insurance? Why not build a system that could take on some of the more routine tasks that confront underwriters everywhere?
RGA’s approach is simple: we are looking to help underwriters to do more by applying AI that is already firmly established today.