In the United States, term products generally include an option to convert to a permanent policy within a specified period of time, and additional underwriting is usually not required to exercise this option.
When evaluating coverage options, policyholders can either choose to convert their term policies into permanent policies or go through underwriting to secure a new policy. Rooted within those converted polices, however, exists an element of and opportunity for anti-selection.
To understand policyholder behavior as it relates to term conversions, RGA was engaged by the Society of Actuaries (SOA) to research the term conversion experience with a focus on term-to-perm conversion rates and the mortality experience of those who did convert. To gain additional insight into those term conversion behaviors, RGA conducted a survey of the market, traditional experience studies, as well as a predictive analysis.
While all three approaches provided improved insights into the understanding of the term conversion parameters and experience, below we focus exclusively on the predictive findings.
Predictive Analytics
Predictive analytics uses a multivariate approach to further analyze policyholders’ behavior in order to answer the following questions:
- Conversion rate model – What drives policyholders to convert policies in the term period?
- Post-conversion point-in-scale mortality model – How does post-conversion mortality differ from term mortality experience?
- Post-conversion lapse model – What drives policyholders to lapse the policies during the post-conversion period?
With a multivariate approach, one can disentangle the separate impacts of numerous variables, thereby isolating the effect of one particular variable from other contributing variables. These effects can be difficult to see using a univariate analysis.
Key Findings
Issue Age Impact in the Conversion Rate Model
The impact of issue age changes throughout the life of a policy. In early durations, although the difference is small, younger issue ages drive comparatively higher conversion rates. In later durations, the older the issue age, the higher the conversion rate. This is more evident in the shock conversion rate in duration 10 shown in Chart 1.
Chart 1:
Illustrated Model Prediction by Issue Age Group
For a sample cohort
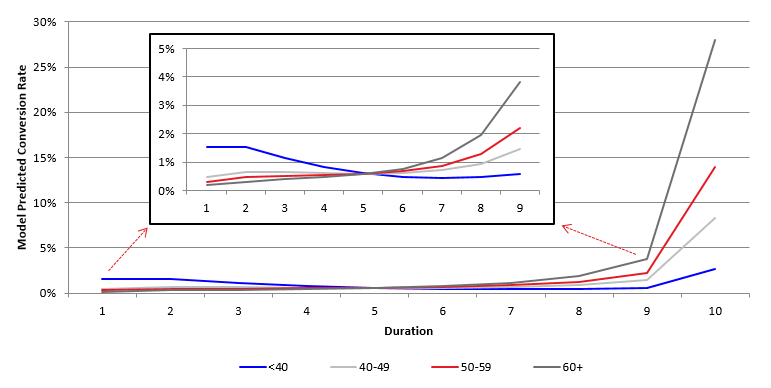
Issue Age Impact in the Post-Conversion Point-in-Scale Mortality Model
The model reveals that the under-40 issue age group has the highest predicted point-in-scale mortality. For all of the age groups, the predicted point-in-scale mortality decreases as duration from conversion increases, as seen in Chart 2. This finding differs from the traditional analysis because the model is able to remove the correlated impact from face amount and isolate the impact of issue age on point-in-scale mortality.
Chart 2:
Illustrated Predicted Point-in-Scale Mortality Ratio by Issue Age Group
For a sample cohort
Duration Impact in the Post-Conversion Lapse Model
The model reveals in Chart 3 that the lapse rate demonstrates a similar trend by duration since conversion. Furthermore, the lapse rate is lower for those policies that convert later. In other words, late converters tend to hold on to the converted policies longer than early converters do.
Predictive Modeling Best Practice
Successful predictive modeling requires suitable models, good functional forms and rigorous validation. Predictive modeling is similar to other actuarial activities in that understanding the data is key and having a firm grasp of the data allows one to interpret the model results more accurately. Insights about the business being analyzed are also extremely valuable when building a meaningful predictive model.
Relying solely on a statistical approach with complex models does increase the predictive accuracy of the model, but it can also make model interpretation and implementation more difficult. One of the major objectives when modeling for actuarial applications is to achieve a balance of complexity, accuracy and simplicity, which calls for a combination of business knowledge and modeling expertise.
Predictive models allow the insurance industry to gain new insights into various aspects of the business. RGA is at the forefront of research and analysis with the expertise and resources to help insurers capitalize on the full potential of predictive analytics.